How AI and content-adaptive encoding are changing video streaming efficiency
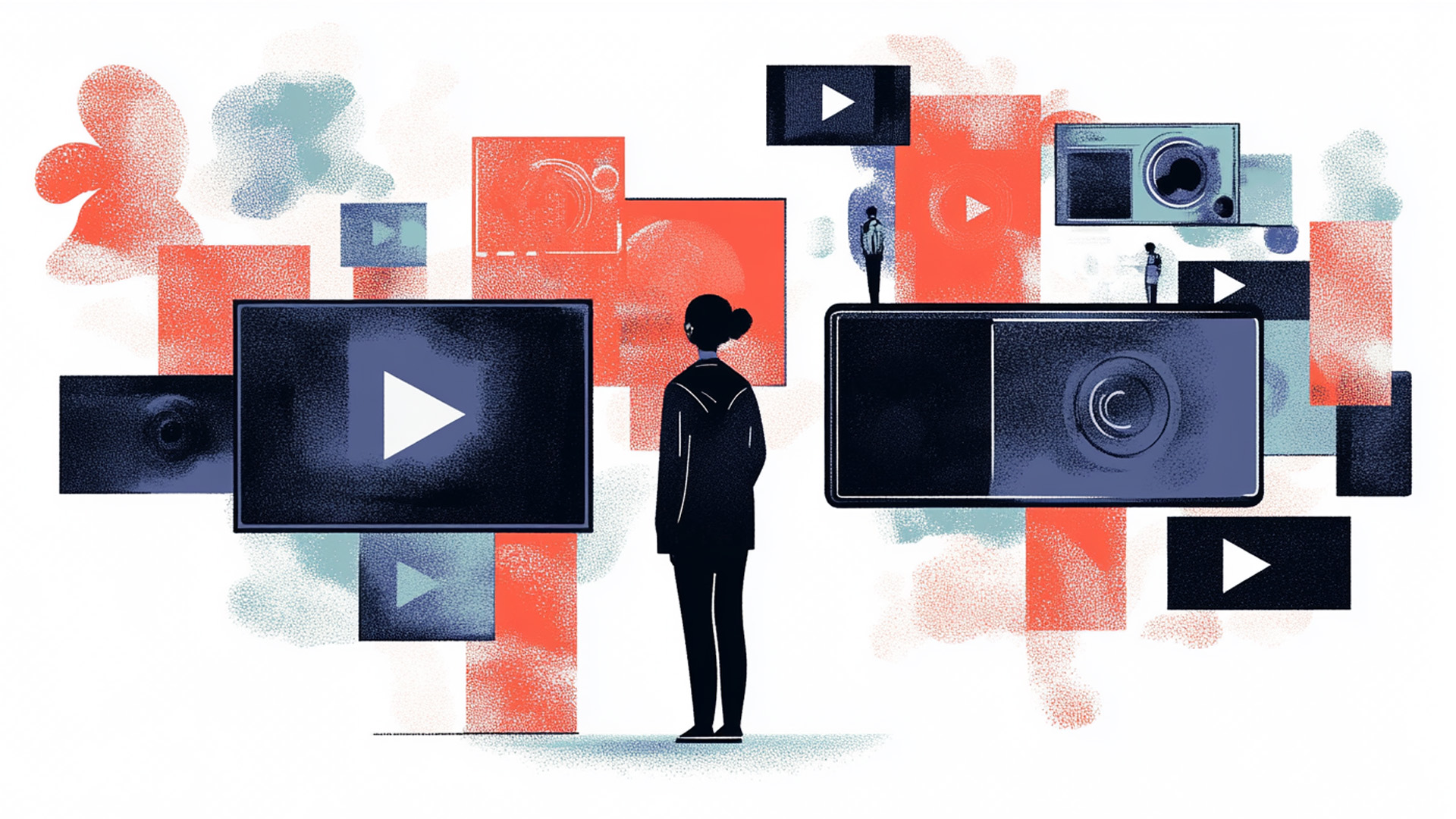
Subscribe to NCS for the latest news, project case studies and product announcements in broadcast technology, creative design and engineering delivered to your inbox.
The explosive growth of video streaming has placed increasing pressure on networks and service providers to deliver high-quality content efficiently. As global video consumption continues to rise, media companies are adopting artificial intelligence (AI) and Content-Adaptive Encoding (CAE) to optimize bandwidth usage, reduce costs, and enhance viewer experiences. By dynamically adjusting encoding parameters based on video complexity, CAE has significantly improved streaming efficiency — allowing platforms to deliver high-quality video while minimizing data consumption.
The evolution of content-adaptive encoding
Between 2015 and 2018, Netflix pioneered content-adaptive encoding, achieving over 30% bitrate reduction without degrading video quality, as measured by the Video Multi-Method Assessment Fusion (VMAF) metric. Unlike traditional encoding methods that apply uniform compression settings across all content, CAE dynamically optimizes encoding settings for each video segment through an exhaustive search process. By analyzing spatial and temporal complexities, CAE ensures that simpler scenes receive lower bitrates, while complex, high-motion sequences are allocated higher bitrates to preserve quality.
While this early approach to CAE was highly effective, it was also computationally expensive and difficult to scale. However, advancements in heuristic CAE solutions and AI-driven encoding methods have since emerged, offering near-optimal results with significantly lower computational costs and enabling CAE for live streaming as well. These innovations have made CAE more accessible to a broader range of streaming providers, helping them lower storage and transmission costs without sacrificing visual fidelity.
AI-powered encoding and real-time adaptation
The integration of machine learning-based encoding frameworks has further refined CAE, enabling real-time encoder parameter optimization. New AI-driven models predict encoding settings — such as bitrate, constant rate factor (CRF), and video buffer verifier (VBV) parameters — by analyzing frame-by-frame content complexity.
For instance, VisualOn Optimizer, a machine learning-based universal CAE framework, utilizes spatial and temporal feature extraction to classify video segments and determine the most efficient encoding parameters. During training, encoder settings are optimized using Particle Swarm Optimization (PSO) to match the texture complexity of specific video categories. During real-time encoding, the classifier dynamically adjusts parameters based on video texture features and target quality metrics, such as VMAF. This real-time feedback loop ensures that every video segment is encoded at the optimal balance of quality and bitrate.
Industry adoption and real-world impact
Leading streaming platforms, including Netflix, YouTube, and Amazon Prime Video, have embraced CAE and AI-driven encoding techniques to optimize content delivery. According to a Netflix study, AI-powered encoding strategies have led to a 20%–30% reduction in data usage while maintaining the same perceptual video quality. Similarly, Google’s AI-enhanced VP9 and AV1 codecs have been shown to reduce streaming bandwidth by up to 30%, making high-quality video more accessible even in bandwidth-constrained regions.
For live streaming services — such as Twitch, YouTube Live, and sports broadcasting platforms — AI-powered encoding dynamically adapts to real-time network conditions. By continuously optimizing compression levels based on available bandwidth and device capabilities, rebuffering rates have been reduced by up to 50%, improving the overall viewer experience.
The future of AI-driven video encoding
As video streaming continues to dominate digital content consumption, AI-powered Content-Adaptive Encoding is proving to be a game-changer in video delivery efficiency. By reducing bandwidth requirements while maintaining high video quality, CAE is helping streaming providers lower costs, improve scalability – especially for large live events, and enhance viewer satisfaction. The ongoing development of AI-driven one-pass encoding solutions and real-time adaptive encoding frameworks will further refine streaming efficiency, ensuring that high-quality video reaches audiences worldwide with minimal resource consumption.
With the convergence of machine learning, AI-based compression, and real-time optimization, the future of video streaming lies in smarter, more efficient encoding strategies. As these technologies continue to evolve, they will redefine how media is delivered, making high-quality video more accessible and sustainable in an increasingly data-intensive world.
Subscribe to NCS for the latest news, project case studies and product announcements in broadcast technology, creative design and engineering delivered to your inbox.
tags
Artificial Intelligence, Content Distribution, content-adaptive encoding, encoders and decoders, VisualOn, VisualOn Optimizer, Yang Cai
categories
Broadcast Automation, Content Delivery and Storage, Featured, Playout & Video Transmission, Streaming, Thought Leadership, Voices