Column: Simplifying live broadcast operations using AI and machine learning
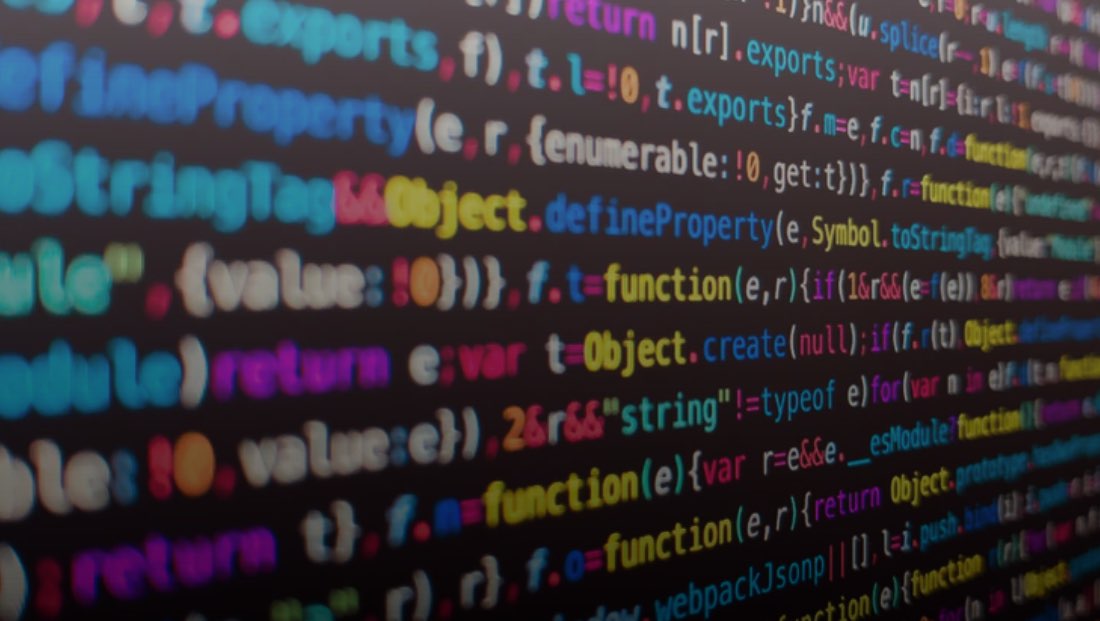
Subscribe to NCS for the latest news, project case studies and product announcements in broadcast technology, creative design and engineering delivered to your inbox.
Pulling back the curtain on AI and machine learning in live broadcast workflows
Artificial Intelligence and machine learning are seen as pillars of the next generation of technological advancement in broadcast media for a variety of reasons, including the ability to sift through mountains of data while identifying anomalies, spotting trends and alerting users to potential problems before they occur without the need for human intervention. With the more data they ingest these models improve over time, meaning the more ML models utilized across a variety of applications, the faster and more complex the insights derived from these tools become.
But to truly understand why machine learning provides enormous value for broadcasters, let’s break it down into use cases and components within broadcast media where AI and ML can have the greatest impact.
Setting the stage: Issues every broadcaster wants to avoid
Imagine a live sporting event stops streaming, or that frames start dropping for no apparent reason. Viewers are noticing quality problems and starting to complain. Technicians are baffled and customers may have just missed the play of the year. Revenue therefore takes a hit and executives want to know what is to blame.
These are situations every broadcaster wants to avoid, and in these tense moments there is no time to lose – viewers are flipping to other services and ad revenue is being lost by the second. What went wrong? Who or what is to blame and how can we get this back up and running immediately, while mitigating this risk in the future? Modern broadcasters need to know before problems happen – not be caught in a crisis trying to pick up the pieces after an incident.
How can AI and machine learning reduce complexity?
The promise of our interconnected world means video workflows are interacting, intertwining, and integrating in new ways every day, simultaneously increasing information sharing, agility and connectivity while producing increasingly complex challenges and issues to diagnose. As more on-prem and cloud resources are connected with equipment from different vendors, sources, and partner organizations distributing to new device types, there is an enormous, ever-expanding number of log and telemetry data produced.
As a result, broadcast engineers have more information than they can effectively process. They routinely silence frequent alerts and alarms because with too much data overload it can be impossible to tell what is important and what is not. This inevitably leaves teams overwhelmed and lacking insights.
Advanced analytics and ML can help with these problems by making sense of overwhelming quantities of data, allowing human operators to sift through insignificant clutter and to focus and understand where issues are likely to occur before failures are noticed. Advanced analytics provide media companies the unprecedented opportunity to leverage sophisticated event correlation, data aggregation, deep learning, and virtually limitless applications to improve broadcast workflows. The benefit is to be able to do more with less, to innovate faster than the competition and prepare for the future – both by increasing your knowledge base and opening the potential for cost reduction and time savings, honing in on the crucial details behind the data that matters most to both their users and organization.
The critical role of anomaly detection in modern broadcasting
One of the biggest challenges facing broadcast operations engineers is to recognize when things are not working before the viewers’ experience is affected. In a perfect world operators and engineers want to predict outages and identify potential issues ahead of time. Machine learning models can be orchestrated to recognize the normal ranges based on hundreds to thousands of measurements – beyond the ability of a human operator – and alert the operator in real time when a stream anomaly occurs. While this process normally requires monitoring logs on dozens of machines and keeping track of the performance of network links between multiple locations and partners, using ML allows the system to identify patterns in large data sets and helps operators focus only on workflow anomalies – dramatically reducing workload.
Anomaly detection works by building a predictive model of what the next measurements related to a stream will be – for example, the round-trip time of packets on the network or the raw bitrate of the stream – and then determining how different the expected value is from the next measurement. As a tool to sort through normal and abnormal streams, this can be essential, especially when managing hundreds or thousands of concurrent channels. One benefit of anomalous behavior identification would be enabling an operator to switch to a backup link that uses a different network link before a failure occurs.
Anomaly detection can also be a vital component of reducing needless false alarms and reducing time waste. Functionality such as customizable alerting preferences and aggregated health scores generated by threat-gauging data points assist operators to sift through and assimilate data trends so they can focus where they really need to. In addition, predictive and proactive alerting can be orders of magnitude less expensive and allow broadcasters to be able to identify the root causes of instability and failure faster and easier.
The challenge of data collection and value of data aggregation
A major challenge to any analytics system is data collection. When you have a video workflow comprised of machines in disparate data centers running different operating systems and tools, it can be difficult to assimilate and standardize reliable, relevant data that can be used in any AI/ML system. While there are natural data aggregation points in most broadcast architectures – for example if you are using a cloud operations and remote management platform or common protocol stack – this is certainly not a given.
Although standards exist for how video data should be formatted and transmitted, few actually describe how machine data, network measurements, and other telemetry should be collected, transmitted and stored. Therefore it is essential to select a technology partner that sends data to a common aggregation point where it is parsed, normalized and put into a database while supporting multiple protocols to support a robust AI/ML solution.
Realizing the true value behind machine learning
Once you have a method for collecting real-time measurements from your video workflow, you can feed this data into a ML engine to detect patterns. From there you can train the system not only to understand normal operating behavior for anomaly detection, but also to recognize specific patterns leading up to video degradation events. With these patterns determined you can also identify common metadata related to degradation events across systems, allowing you to identify that the degradation event is related to a particular shared network segment.
For example, if a particular ISP in a particular region continues to experience latency or blackout issues, the system learns to pick up on warning signs ahead of time and notifies the engineer before an outage – preventing issues proactively while simultaneously improving root cause identification within your entire ecosystem. Developers can also see that errors are more often observed using common encoder or network hardware settings. Unexpected changes in the structure of the video stream or the encoding quality might also be important signals of impending problems. By observing correlations, ML gives operators key insights into the causes of problems and how to solve them.
Not all issues are avoidable, but ML assisted root cause analysis helps prevent future risk
Predictive analytics, alerts and correlations are useful for automated failure prediction and alerting, but when all else fails, ML models can also be used to help operators concentrate on areas of concern following an outage, making retrospective analysis much easier and faster via root cause analysis.
With workflows that consist of dozens of machines and network segments, it is inherently difficult to know where to look for problems. However, ML models, as we have seen, provide trend identification and help visualize issues using data aggregation. Even relatively straightforward visualizations of how a stream deviates from the norm are incredibly valuable, whether in the form of historical charts, customizable reports or questions as simple as how a particular stream compares to a similar recent stream.
Machine learning points to the future of successful broadcast workflows
Leveraging AI and ML to improve operational efficiency and quality provides a powerful advantage while preparing broadcasters for the future of live content delivery over IP. Selecting the right vendor for system monitoring and orchestration that integrates AI and ML capabilities can help your organization make sense of the vast amounts of data being sent across the media supply chain and be a powerful differentiator.
As experiments to test hypotheses are essential to the traditional learning process, the same goes for ML models. Building, training, deploying, and updating ML models are inherently complex, meaning providers in cooperation with their users must continue to iterate, compare results, and adjust accordingly to understand the why behind the data, improving root cause analysis and the customer experience.
Machine learning presents an unprecedented opportunity for sophisticated event correlation, data aggregation, deep learning, and virtually unlimited applications across broadcast media operations as it evolves exponentially year to year. As models become more informed and interconnected, problem solving and resolution technology based on deep learning and AI will become increasingly essential tools. Broadcast organizations looking to prepare themselves for such a future would be wise to prepare for this eventuality by choosing the right vendor to integrate AI and ML enabled tools into their workflows.
Subscribe to NCS for the latest news, project case studies and product announcements in broadcast technology, creative design and engineering delivered to your inbox.
tags
Andrew Broadstone, Artificial Intelligence, Automatic Metadata Extraction, Broadcast Workflow, data analytics, machine learning, Metadata, workflow, Zixi
categories
Broadcast Engineering, Featured, IP Based Production, Software, Voices